News
AI News and Accomplishments
April 5, 2024
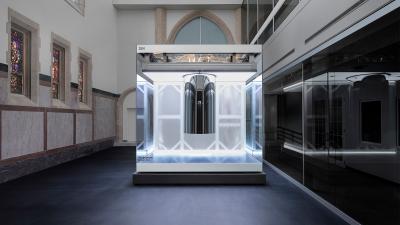
April 4, 2024
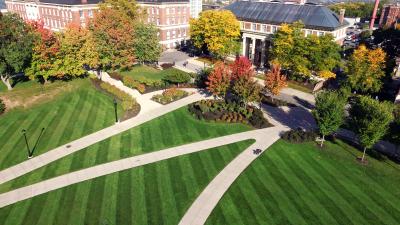
Deadline for submission is Saturday, March 30, 11:59 PM
Call for Posters: RPI QC Ribbon Cutting and Bicentennial Ceremony
The poster session at the upcoming quantum computer ribbon-cutting ceremony and bicentennial ceremony is an opportunity for the RPI community to showcase its computing capabilities. Submissions for posters must include either:
March 18, 2024
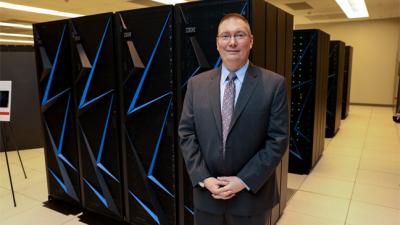
March 5, 2024
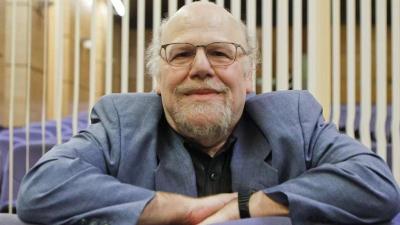
Rensselaer News Feed
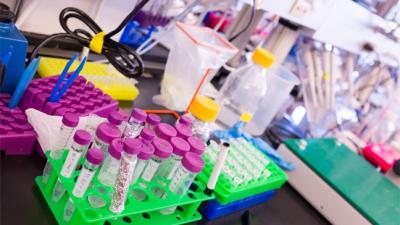
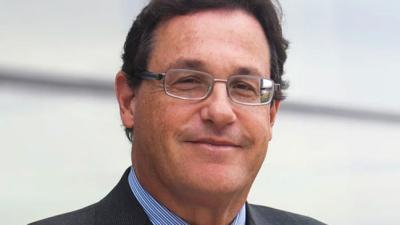
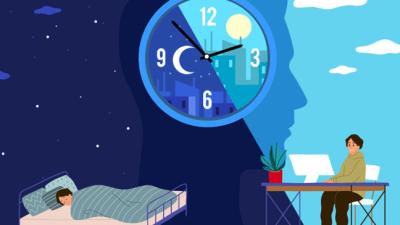
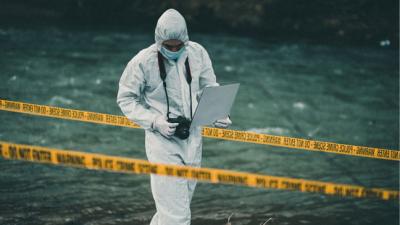
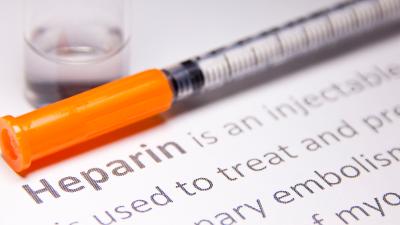